New Tech. The Next Frontier: Generative AI in the Physical World
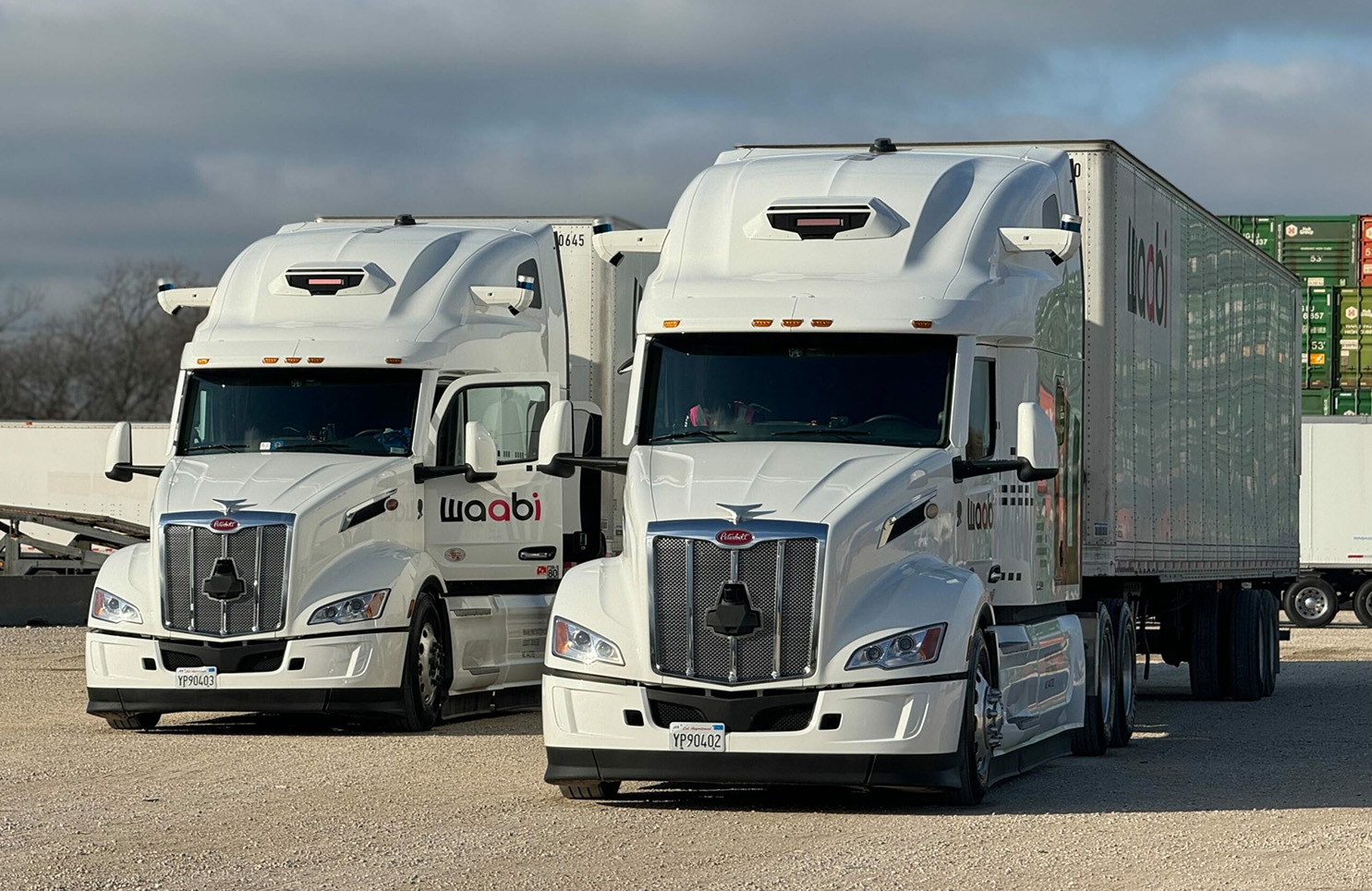
CRAZY Advances in Artificial Intelligence over recent years have been nothing short of remarkable. We’ve witnessed AI ace the notoriously hard Bar exam, produce highly sophisticated and life-like images, audio and videos, as well as transform productivity across many industries. Our experiences within the digital realm are being completely reimagined as AI advances.
This digital world revolution has been fueled by three key ingredients: vast and ever-expanding repositories of large-scale data, breakthroughs in accelerated computing, and major advancements in algorithms and scalable neural network architectures. These elements have enabled the development of foundation models, which are typically trained on massive datasets without human supervision and serve as the backbone for a multitude of generative AI applications.
As extraordinary as these advances are, we’ve still only scratched the surface of AI’s potential. The next leap forward will see generative AI graduate out of the virtual world and move into the physical world where it will interact with humans in their day-to-day lives. However, this new frontier necessitates innovative approaches to building AI systems, as the physical world presents unique challenges that need to be addressed.
Firstly, AI systems operating in the physical world must be able to generalize to a broad range of situations, ensuring robust performance across both common scenarios as well as the longtail of unpredictable ones. Additionally, these systems need to be incredibly efficient and capable of running on edge devices, where computational resources are limited. Further, safety takes on a new meaning when considering AI in the physical world: any system deployed must adhere to rigorous safety standards, that in many cases don’t even exist today, to prevent accidents and ensure trustworthy behavior.
I have spent more than two decades dedicated to inventing new AI technologies that can address these challenges and deliver on the enormous potential of AI in the physical world. With a focus on self-driving, I have pursued a vision of a single AI system that can learn end-to-end to perform highly complex tasks that can be executed fully autonomously and in a provably safe manner.
By “provably safe” I mean that, before deployment in the physical world, fully autonomous systems need to be validated and verified, with robust scientific evidence forming the safety case. In the self-driving industry, in particular, there’s a need to go far beyond the current practice which is built on accumulating miles of real-world driving as a measure of deployment readiness. While the volume of these miles is in the millions, it is nowhere near what would be required to provide the rigorous evidence necessary for a comprehensive safety case.
Three years ago I started Waabi to turn my vision into a tangible product that would introduce this incredible technology to the world. I chose to focus first on autonomous trucking. Not only is the logistics industry facing considerable inefficiencies across supply chains and a looming shortage of truck drivers, but the job itself can be grueling and safety continues to be a major concern. Autonomous trucks have tremendous potential to address these challenges, significantly increasing asset utilization, reducing carbon emissions, improving road safety, and positively reimagining the career outlook and quality of life for truck drivers.
Core to our approach at Waabi has been the development of a groundbreaking new generation of foundation models that are uniquely capable of perceiving the world, creating interpretable abstractions, and then using these abstractions to reason in the same way humans do, in order to find the best possible action to perform. These models are the backbone of our virtual driver — the Waabi Driver.
A system like this, capable of human-like reasoning, has astounding abilities to generalize across the unknown, which not only results in safer behavior but also unlocks an unprecedented data efficiency in training. This stands in contrast to other end-to-end approaches in self-driving that rely on blackbox-like architectures which lack interpretability, and do not have the ability to reason. These models require extraordinary amounts of training data, collected from humans driving within massive connected fleets, which are then provided as examples for the network to imitate. Even then this approach falls short in providing sufficient exposure to safety-critical situations that are crucial for ensuring the reliability of self-driving systems.
This raises two important questions: how can you prove that a system is safer than humans when many incidents, particularly safety-critical situations with potentially catastrophic consequences, happen very infrequently? And, how do you teach a system the right course of action in these situations? It’s not like you can intentionally test your system in safety-critical scenarios in the real world—doing so would be highly inappropriate and dangerous.
A second central tenet to our approach at Waabi has been the invention of a revolutionary high-fidelity, closed-loop simulator called Waabi World which is the answer to these open questions. Waabi World automatically generates all the situations a self-driving vehicle might encounter in the real world, and tests the entire autonomy system with near-zero domain gap.
This means that the AI system under test should drive exactly the same way in simulation as it would in the real world when presented with the same situation. Furthermore, Waabi World is a super-intelligent engine that can efficiently create scenarios that, with high probability, make the system under test fail, as well as cover all the possible failures it might have. This provides extraordinary levels of efficiency in testing.
Waabi World also solves the data problem that plagues the broader AI industry by intelligently creating richer, more informative examples than real-world data, massively reducing training and testing times as well as minimizing costs. It is a revolutionary system that has extraordinary positive consequences in the development and deployment of fully autonomous systems — well beyond trucking — dramatically reducing development risk and providing the first solution to perform a rigorous scientific safety case.
In only three years since launch, the Waabi Driver is already driving autonomously on public roads in Texas, regularly completing commercial runs for some of the largest shippers in North America. We have set a new pace and standard for self-driving companies with our revolutionary foundation model that, paired with Waabi World, the world’s most advanced generative AI powered simulator, enables a safer, more efficient solution that is highly performant and scalable from day one.
By Raquel Urtasun Waabi CEO